Hadoop 2.0 (YARN) Framework - The Gateway to Easier Programming for Hadoop Users
Apache Hadoop is the foundation for all the big data architectures. This article compares and explains difference between Hadoop 1.0 and Hadoop 2.0 YARN.
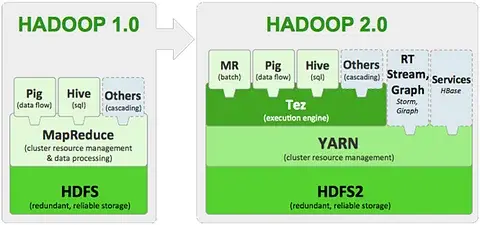
With a rapid pace in evolution of Big Data, its processing frameworks also seem to be evolving in a full swing mode. Hadoop (Hadoop 1.0) has progressed from a more restricted processing model of batch oriented MapReduce jobs to developing specialized and interactive processing models (Hadoop 2.0). With the advent of Hadoop 2.0, it is possible for organizations to create data crunching methodologies within Hadoop which were not possible with Hadoop 1.0 architectural limitations. In this piece of writing we provide the users an insight on the novel Hadoop 2.0 (YARN) and help them understand the need to switch from Hadoop 1.0 to Hadoop 2.0.
Airline Dataset Analysis using Hadoop, Hive, Pig and Athena
Downloadable solution code | Explanatory videos | Tech Support
Start ProjectTable of Contents
Evolution of Hadoop 2.0 (YARN) -Swiss Army Knife of Big Data
With the introduction of Hadoop in 2005 to support cluster distributed processing of large scale data workloads through the MapReduce processing engine, Hadoop has undergone a great refurbishment over time. The result of this is a better and advanced Hadoop framework that does not merely support MapReduce but renders support to various other distributed processing models also.
The huge data giants on the web such as Google, Yahoo and Facebook who had adopted Apache Hadoop had to depend on the partnership of Hadoop HDFS with the resource management environment and MapReduce programming. These technologies collectively enabled the users to manage processes and store huge amounts of semi-structured, structured or unstructured data within Hadoop clusters. Nevertheless there were certain intrinsic drawbacks with Hadoop MapReduce pairing. For instance, Google and other users of Apache Hadoop had various alluding issues with Hadoop 1.0 of not having the ability to keep track with the flood of information that they were collecting online due to the batch processing arrangement of MapReduce.
New Projects
Introduction to Hadoop YARN (Hadoop 2.0)
Hadoop 2.0 popularly known as YARN (Yet another Resource Negotiator) is the latest technology introduced in Oct 2013 that is being used widely nowadays for processing and managing distributed big data.
Get FREE Access to Data Analytics Example Codes for Data Cleaning, Data Munging, and Data Visualization
Hadoop YARN is an advancement to Hadoop 1.0 released to provide performance enhancements which will benefit all the technologies connected with the Hadoop Ecosystem along with the Hive data warehouse and the Hadoop database (HBase). Hadoop YARN comes along with the Hadoop 2.x distributions that are shipped by Hadoop distributors. YARN performs job scheduling and resource management duties devoid of the users having to use Hadoop MapReduce on Hadoop Systems.
Hadoop YARN has a modified architecture unlike the intrinsic characteristics of Hadoop 1.0 so that the systems can scale up to new levels and responsibilities can be clearly assigned to the various components in Hadoop HDFS.
Need to Switch from Hadoop 1.0 to Hadoop 2.0 (YARN)
The foremost version of Hadoop had both advantages and disadvantages. Hadoop MapReduce is a standard established for big data processing systems in the modern era but the Hadoop MapReduce architecture does have some drawbacks which generally come into action when dealing with huge clusters.
Here's what valued users are saying about ProjectPro
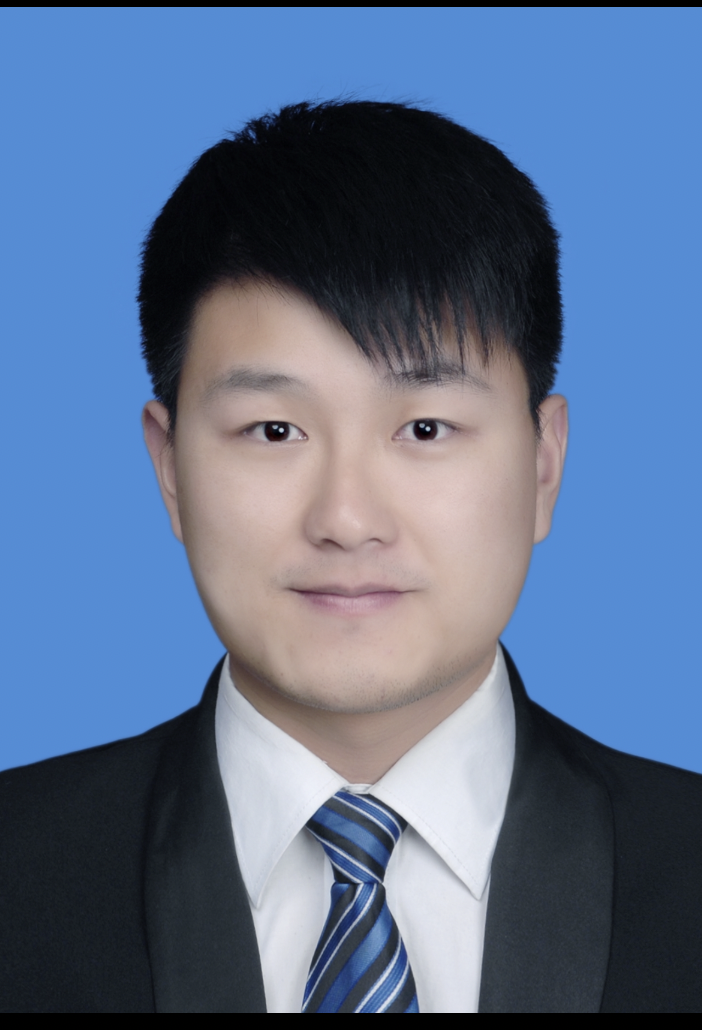
Jingwei Li
Graduate Research assistance at Stony Brook University
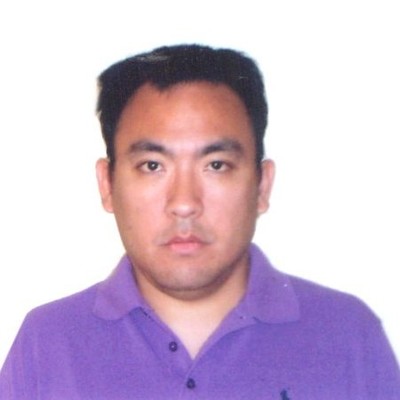
Ray han
Tech Leader | Stanford / Yale University
Not sure what you are looking for?
View All ProjectsLimitations of Hadoop 1.0
Issue of Availability:
Hadoop 1.0 Architecture had only one single point of availability i.e. the Job Tracker, so in case if the Job Tracker fails then all the jobs will have to restart.
Issue of Scalability:
The Job Tracker runs on a single machine performing various tasks such as Monitoring, Job Scheduling, Task Scheduling and Resource Management. In spite of the presence of several machines (Data Nodes), they were not being utilized in an efficient manner, thereby limiting the scalability of the system.
Cascading Failure Issue:
In case of Hadoop MapReduce when the number of nodes is greater than 4000 in a cluster, some kind of fickleness is observed. The most common kind of failure that was observed is the cascading failure which in turn could cause the overall cluster to deteriorate when trying to overload the nodes or replicate data via network flooding.
Learn to Manage and Schedule Hadoop Jobs with Oozie Workflow Scheduler
Multi-Tenancy Issue:
The major issue with Hadoop MapReduce that paved way for the advent of Hadoop YARN was multi-tenancy. With the increase in the size of clusters in Hadoop systems, the clusters can be employed for a wide range of models.
Hadoop MapReduce devotes the nodes of the cluster in the Hadoop System so that they can be repurposed for other big data workloads and applications. Nevertheless, with Big Data and Hadoop, ruling the data processing applications for cloud deployments, the number of nodes in the cluster is likely to increase and this issue is addressed with a switch from 1.x to 2.x.
This is not just the end of the limitations coming from Hadoop MapReduce apart from the above mentioned issues there were several other concerns addressed by Hadoop programmers with version 1.0 such as inefficient utilization of the resources, hindering constraints in running any other Non-MapReduce applications, running ad-hoc queries, carrying out real time analysis and limitations in running the message passing approach.
Get More Practice, More Big Data and Analytics Projects, and More guidance.Fast-Track Your Career Transition with ProjectPro
Understanding the Differences between the Components of Hadoop 1.0 and Hadoop 2.0
The Hadoop 1.0 or the so called MRv1 mainly consists of 3 important components namely:
1) Resource Management:
This is an infrastructure component that takes care of monitoring the nodes, allocating the resources and scheduling various jobs.
2) Application Programming Interface (API):
This component is for the users to program various MapReduce applications.
3) Framework:
This component is for all the runtime services such as Shuffling, Sorting and executing Map and Reduce processes.
The major difference with Hadoop 2.0 is that, in this next generation of Hadoop the cluster resource management capabilities are moved into YARN.
YARN
YARN has taken an edge over the cluster management responsibilities from MapReduce, so that now MapReduce just takes care of the Data Processing and other responsibilities are taken care of by YARN.
Hadoop 2.0 (YARN) and Its Components
In Hadoop 2.0, the Job Tracker in YARN mainly depends on 3 important components
1. Resource Manager Component:
This component is considered as the negotiator of all the resources in the cluster. Resource Manager is further categorized into an Application Manager that will manage all the user jobs with the cluster and a pluggable scheduler. This is a relentless YARN service that is designed for receiving and running the applications on the Hadoop Cluster. In Hadoop 2.0, a MapReduce job will be considered as an application.
2. Node Manager Component:
This is the job history server component of YARN which will furnish the information about all the completed jobs. The NM keeps a track of all the users’ jobs and their workflow on any particular given node.
3. Application Master Component (aka User Job Life Cycle Manager):
This is the component where the job actually resides and the Application Master component is responsible for managing each and every Map Reduce job and is concluded once the job completes processing.
A Gist on Hadoop 2.0 Components
RM-Resource Manager
1.It is the global resource scheduler
2.It runs on the Master Node of the Cluster
3.It is responsible for negotiating the resources of the system amongst the competing applications.
4.It keeps a track on the heartbeats from the Node Manager
NM-Node Manager
1.Node Manager communicates with the resource manager.
2.It runs on the Slave Nodes of the Cluster
AM-Application Master
1.There is one AM per application which is application specific or framework specific.
2.The AM runs in Containers that are created by the resource manager on request.
companies that are currently using Hadoop, will establish Hadoop 2.0 as a platform for creating applications and manipulating data for more effectively and efficiently.
Build an Awesome Job Winning Project Portfolio with Solved End-to-End Big Data Projects
YARN is the elephant sized change that Hadoop 2.0 has brought in but undoubtedly there are lots of challenges involved as companies migrate from Hadoop 1.0 to Hadoop 2.0 however the basic changes to the MR framework will have greater usability level for Hadoop in the upcoming big data scenarios. Hadoop 2.0 being more isolated and scalable over the earlier version, it is anticipated that soon there will be several novel tools that will get the most out of the new features in YARN (Hadoop 2.0).
Related Posts
How much Java is required to learn Hadoop?
Top 100 Hadoop Interview Questions and Answers
Difference between Hive and Pig - The Two Key components of Hadoop Ecosystem
About the Author
ProjectPro
ProjectPro is the only online platform designed to help professionals gain practical, hands-on experience in big data, data engineering, data science, and machine learning related technologies. Having over 270+ reusable project templates in data science and big data with step-by-step walkthroughs,